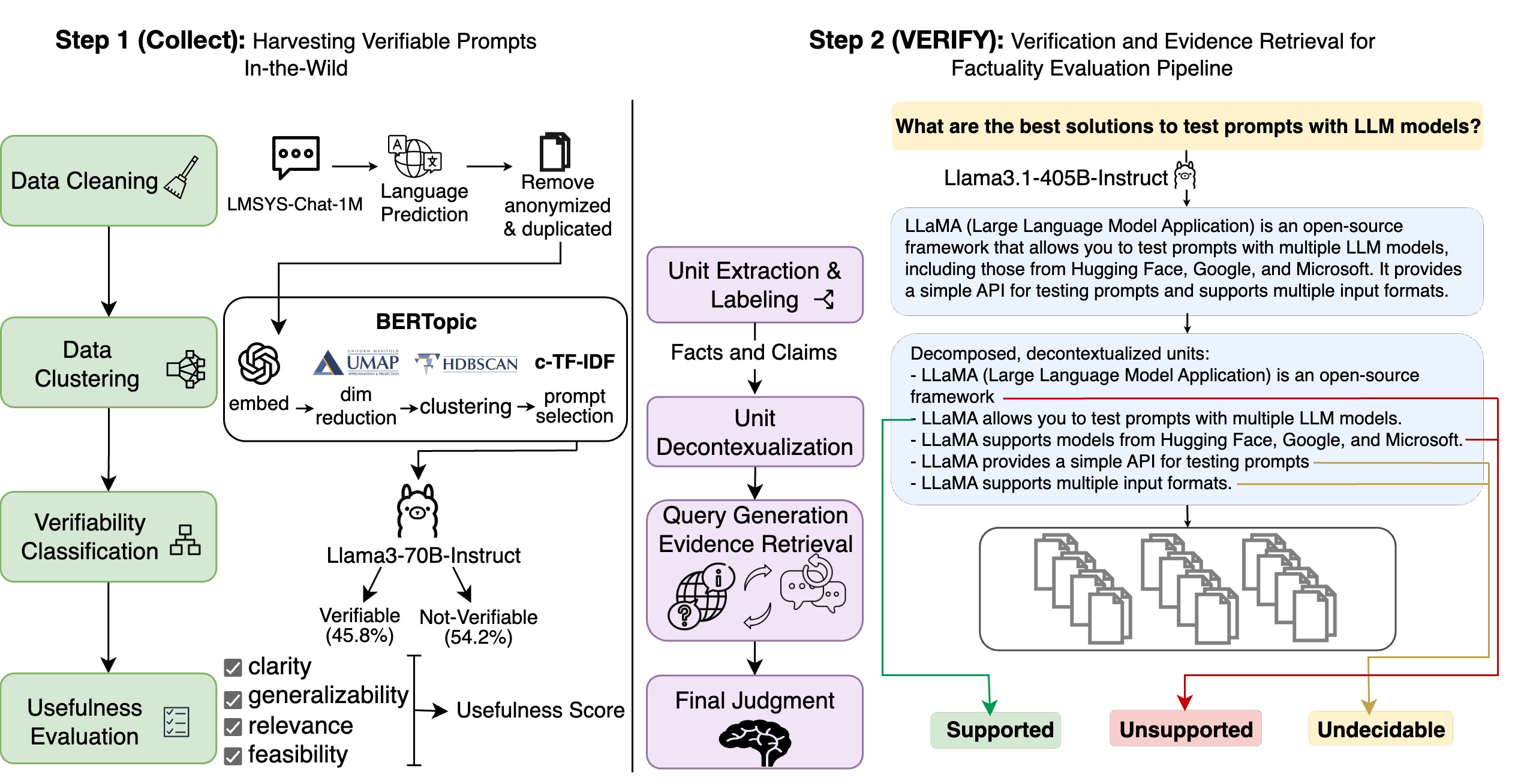
Motivation
The rapid adoption of language models (LMs) in various applications has highlighted the critical need for maintaining factual accuracy across a wide range of topics. As these models become increasingly integrated into our daily lives, the challenge of ensuring their reliability and truthfulness grows more pressing. This motivated the development of FACTBENCH, a dynamic benchmark designed to evaluate the factuality of LMs in real-world scenarios, addressing the limitations of existing static and narrow-scope evaluation methods.
Task
Our task was to create a comprehensive and updatable benchmark for assessing the factual accuracy of language models in diverse, real-world contexts. This involved developing a pipeline to identify "hallucination prompts" - queries that tend to elicit incorrect or inconclusive responses from LMs. We needed to design an effective method for evaluating the factuality of LM-generated content, considering the verifiability of statements and the nuances of different content types.
Action
We developed VERIFY, a Verification and Evidence RetrIeval for FactualitY evaluation pipeline, to systematically assess LM responses. This involved extracting content units from model outputs, classifying them by type, and evaluating verifiable units against web-based evidence. We implemented an interactive query generation and evidence retrieval technique to enhance the quality of fact-checking. Using this pipeline, we curated FACTBENCH, a dataset of 1,000 prompts across 150 fine-grained topics, categorized into different difficulty tiers based on LM performance.
Results
Our work resulted in the creation of FACTBENCH, the first dynamic and in-the-wild factuality evaluation benchmark for language models. This benchmark not only captures emerging factuality challenges in real-world LM interactions but is also regularly updated with new prompts. Through extensive evaluation of widely-used LMs, including GPT, Gemini, and Llama3.1 family models, we uncovered key insights into their factual accuracy across different difficulty levels. Notably, VERIFY achieved the highest correlation with human judgments compared to existing factuality evaluation methods, underscoring its effectiveness in assessing LM factuality.