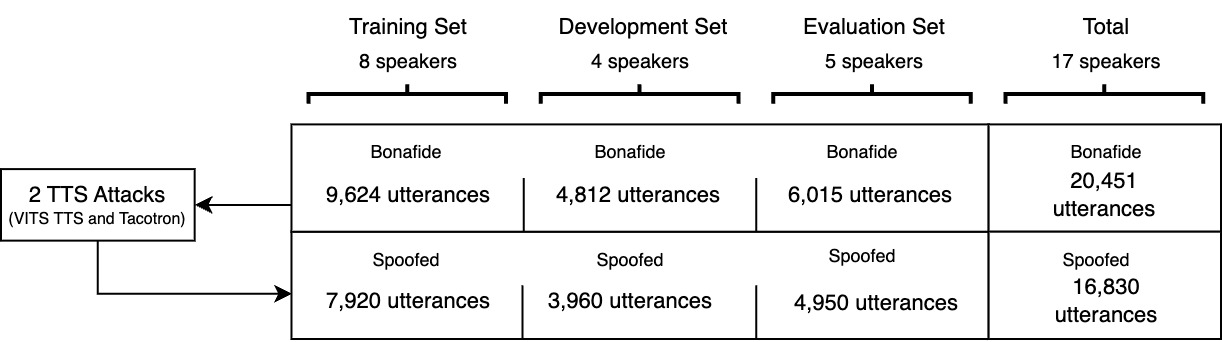
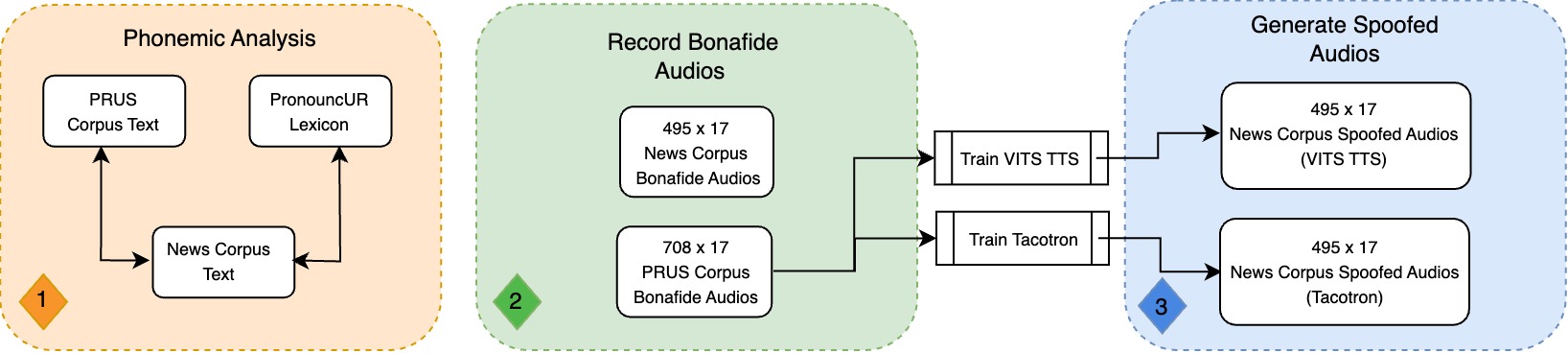
Motivation
The proliferation of deepfake audio, particularly in low-resource languages like Urdu, poses significant challenges for automatic speaker verification systems and has the potential to spread misinformation. Existing datasets for deepfake detection primarily focus on high-resource languages, leaving a critical gap in resources for languages like Urdu. This research aims to address this deficiency by creating a comprehensive Urdu deepfake audio dataset. The creation of synthetic datasets is crucial for developing robust detection models, but it must be done carefully to ensure the dataset's quality and representativeness.
Task
We set out to construct and evaluate a specialized Urdu deepfake audio dataset for deepfake detection. Our goal was to create a resource that would enable the training of robust detection models for Urdu, a low-resource language, while ensuring phonemic cover and balance comparable to established Urdu corpora. The task involved not only generating synthetic data but also rigorously evaluating its quality and trustworthiness to ensure its utility in real-world applications.
Action
We developed a dataset comprising 20,451 utterances of bonafide audio and 16,830 utterances of deepfake audio1 . The deepfake samples were generated using two advanced text-to-speech models: Tacotron and VITS TTS. We conducted rigorous phonemic analysis to ensure the dataset's linguistic representativeness, comparing it with established Urdu corpora such as PRUS and PronouncUR. The phonemic analysis revealed strong correlations with Spearman's Rank Correlation coefficients of 0.977 and 0.958 when compared to PRUS and PronouncUR, respectively1 . Additionally, we performed both qualitative and quantitative evaluations of the dataset, including human evaluation studies and machine learning-based assessments using models like AASIST-L and RawNet2.
Results
Our analysis validated the dataset's utility for training deepfake detection models. The Equal Error Rate (EER) obtained through the AASIST-L model was 0.495 for VITS TTS and 0.524 for Tacotron-generated audios, indicating the challenge in distinguishing between real and fake samples1 . These results highlight the high quality of the synthetic data and the need for advanced countermeasures against audio deepfakes in low-resource languages. Human evaluation showed that approximately one in three fake audio samples were perceived as real, underscoring the potential risks of deepfake audio in spreading misinformation1 . The t-SNE visualization and L2 norm comparisons further demonstrated the spectral similarities between bonafide and deepfake audios, emphasizing the sophistication of the generated synthetic data. These comprehensive evaluations contribute to the trustworthiness of systems trained on this dataset, as they provide a realistic and challenging benchmark for deepfake detection in Urdu.
Samples from the Dataset
Bonafide (spoken/real audio):
TTS Generated (deepfake audio):
Tacotron Generated (deepfake audio):
Media
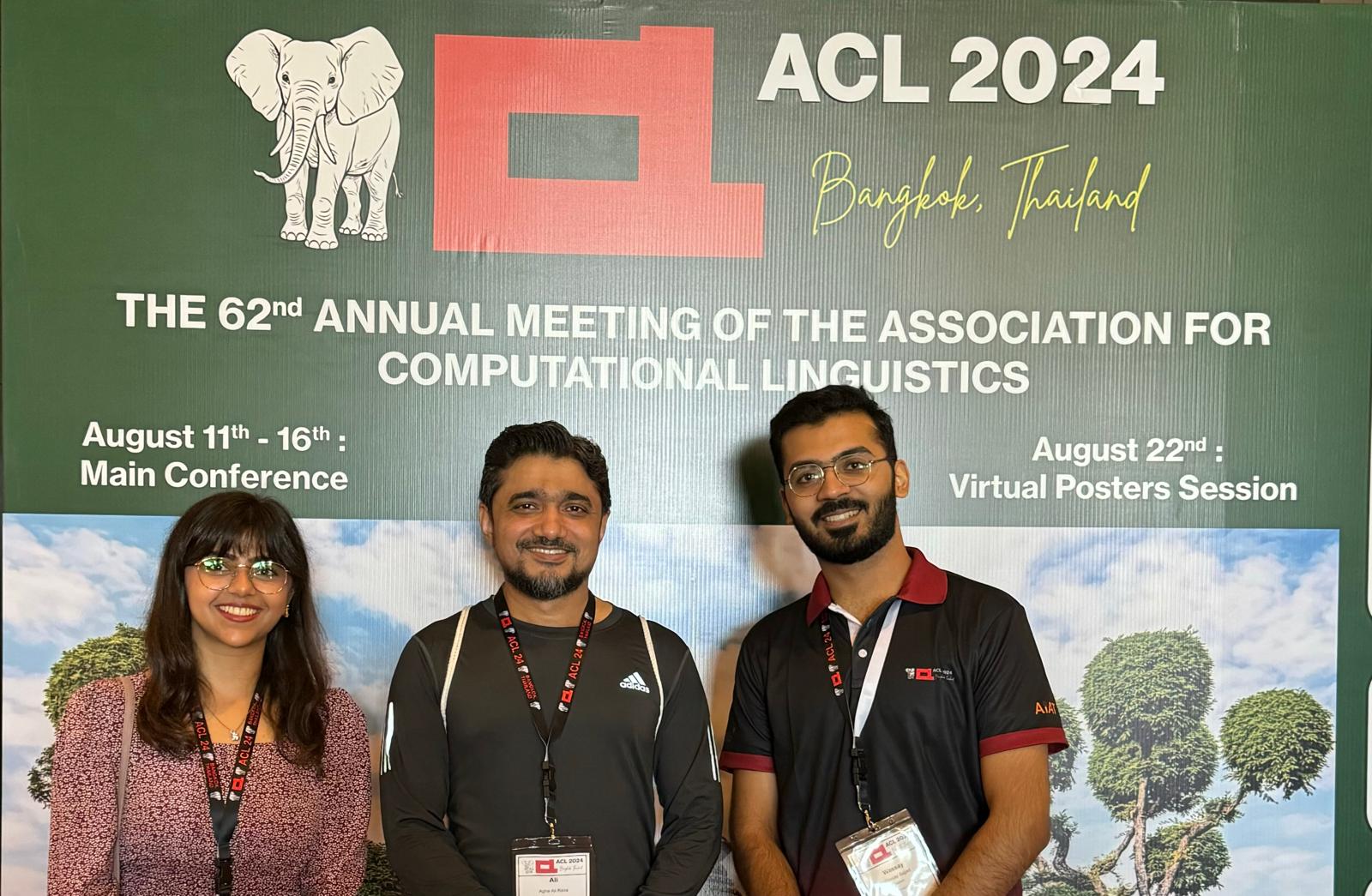
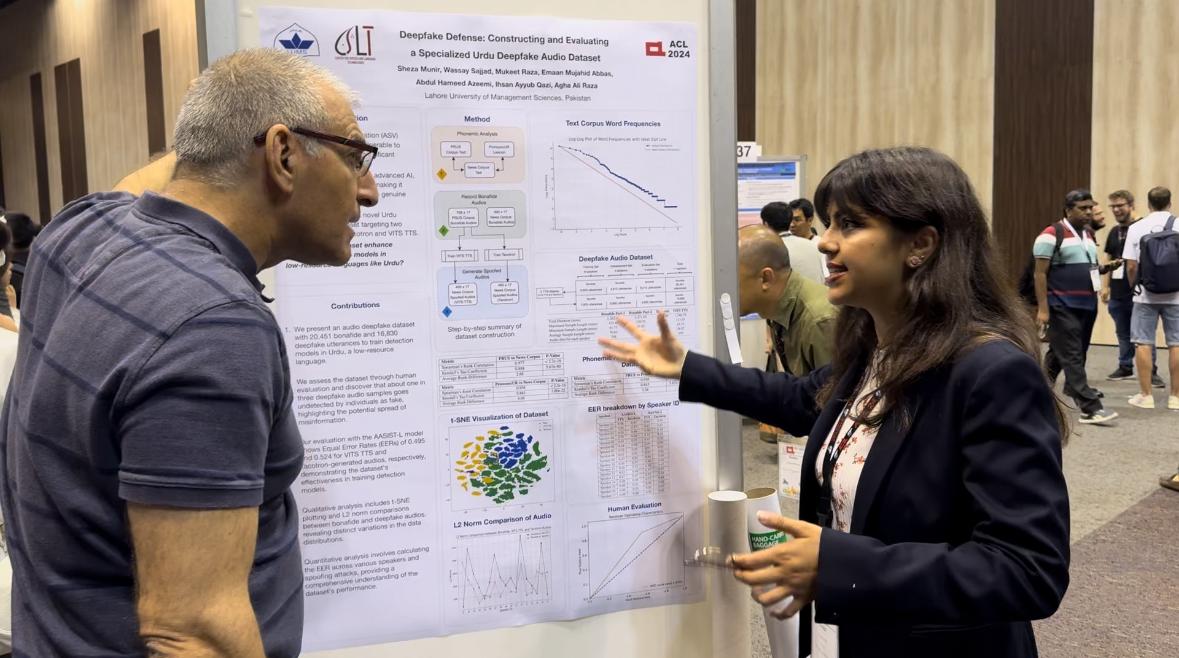